NVIDIA’s New AI: Nature Videos Will Never Be The Same
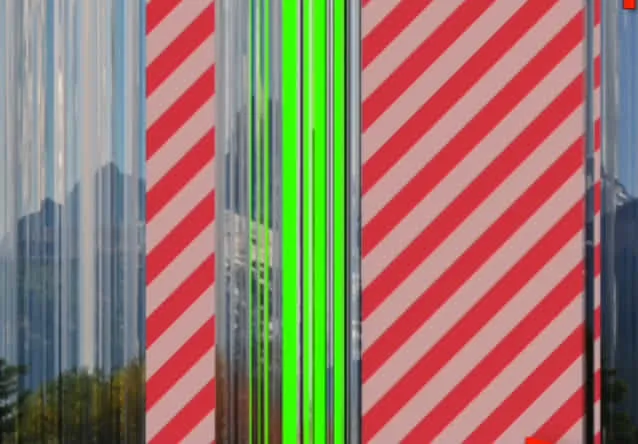
Time-lapse image sequences offer visually compelling insights into dynamic processes that are too slow to observe in real time. However, playing a long time-lapse sequence back as a video often results in distracting flicker due to random effects, such as weather, as well as cyclic effects, such as the day-night cycle. We introduce the problem of disentangling time-lapse sequences in a way that allows separate, after-the-fact control of overall trends, cyclic effects, and random effects in the images, and describe a technique based on data-driven generative models that achieves this goal. This enables us to “re-render” the sequences in ways that would not be possible with the input images alone. For example, we can stabilize a long sequence to focus on plant growth over many months, under selectable, consistent weather.
Our approach is based on Generative Adversarial Networks (GAN) that are conditioned with the time coordinate of the time-lapse sequence. Our architecture and training procedure are designed so that the networks learn to model random variations, such as weather, using the GAN’s latent space, and to disentangle overall trends and cyclic variations by feeding the conditioning time label to the model using Fourier features with specific frequencies.
We show that our models are robust to defects in the training data, enabling us to amend some of the practical difficulties in capturing long time-lapse sequences, such as temporary occlusions, uneven frame spacing, and missing frames.
Keine Kommentare